AI-Powered Decision Engine
for Commodity Buyers & Traders
Datapred helps energy and commodity buyers optimize procurement, manage risks, and make data-driven decisions — all on a single, connected platform.
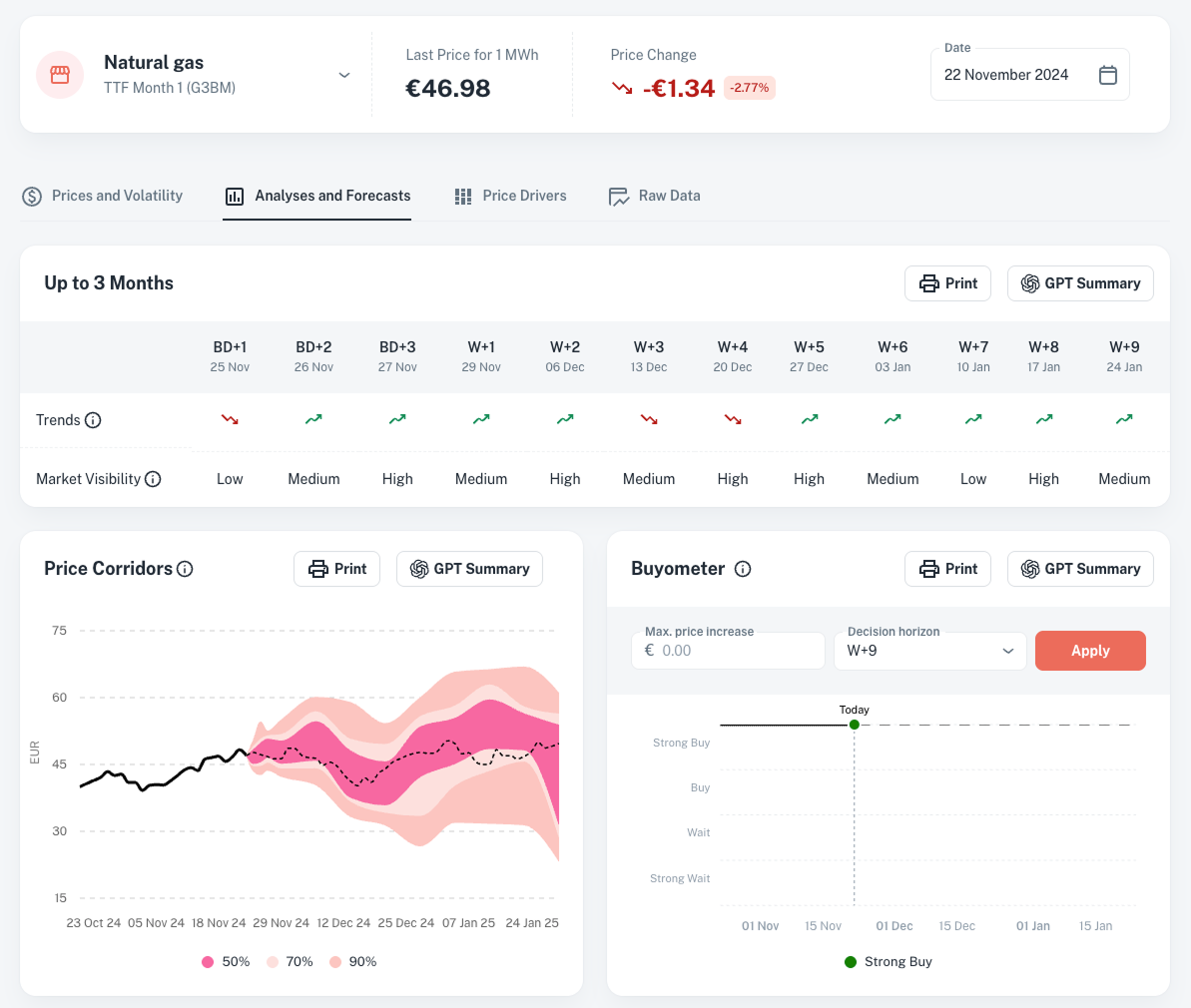
Become the smartest buyer:
Know when and how much to buy,
and save 2-5% on your total procurement cost
How Datapred Works
Optimize your purchasing & hedging strategies with our 3 modules.
First results available in 6-8 weeks, refinable as needed to meet more advanced requirements.

Consolidate and Visualize Energy & Raw Material Consumption

Eliminate spreadsheet chaos - no more juggling 45 Excel files. Save time with a structured, transparent, and actionable data overview.

Centralize and Analyze All Historical Transactions

Boost efficiency and clarity - replace scattered files with a single, intuitive platform offering real-time insights into your procurement history.

Prices & Volatility
Instant Market Insights

Know your market instantly and react proactively.

Analyses & Forecasts
AI-Powered Price Projections

Adapt to market regime changes, monitor prediction accuracy, and assess model performance over time.

Price Drivers
Understand What Drives Your Market

Focus on the most relevant data streams and avoid unnecessary data costs.

Data Stream Selection
Optimize Your Market Intelligence

Ensure that your forecasting models rely on the most relevant and cost-effective data.

Configuration
Design & Test Multiple Strategies

Test all the purchasing / hedging strategies you’ve ever wanted in a safe and protected environment.

Program
AI-Powered Strategy Execution

Gain first-class AI-driven recommendations while keeping full decision-making autonomy, and reduce annual costs by 2-5%.

Benchmarking
Compare & Optimize Performance

Identify and select the most effective purchasing / hedging strategies, and continuously monitor them.
Fit with Your Market
Procurement professionals : gain visibility and reduce costs.
Manage energy procurement with real-time AI-driven insights.
Optimize hedging, forecast demand, and secure supply stability.
Gain visibility into oil-based material markets with real-time AI insights.
Optimize sourcing strategies, anticipate cost fluctuations, and improve contract negotiations.
Stay ahead of agricultural market trends with intelligent analytics.
Forecast seasonal variations, reduce exposure to volatility, and streamline procurement planning.
Take control of metal procurement with dynamic market intelligence.
Respond to price shifts, forecast demand accurately, and ensure supply continuity at the best cost.
Turn Real-Time Data into
Confident, Cost-Saving Decisions
Turn Real-Time Data into Confident, Cost-Saving Decisions.
Helping companies optimize their purchasing strategies, mitigate market risks, and drive better decisions through advanced data insights and forecasting.
Consolidate & Visualize Energy and Raw Material Consumption
Say goodbye to scattered spreadsheets. Get a clear, centralized view of your consumption data and save valuable time.
Discover More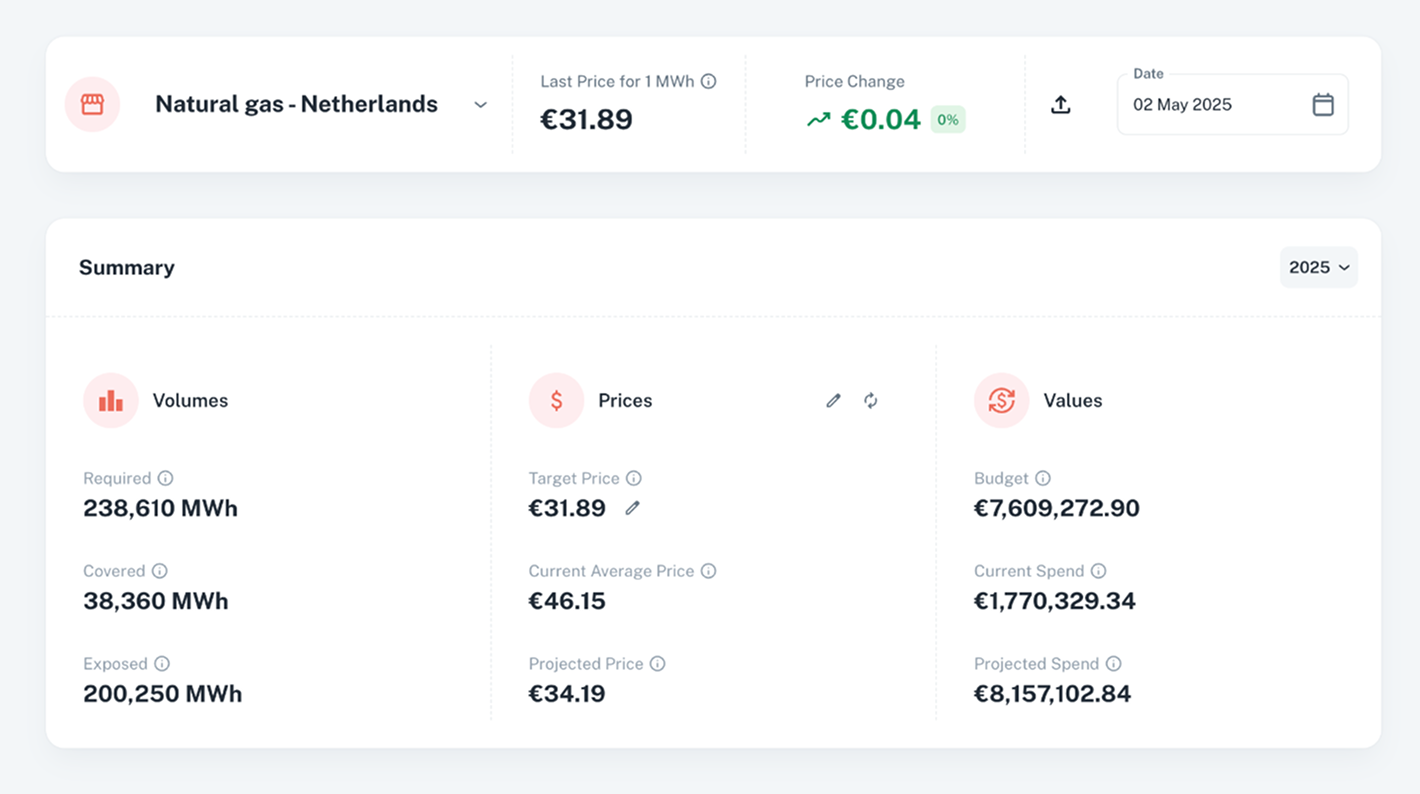
Centralize & Analyze All Historical Transactions
Streamline your processes with one centralized tool delivering instant insights into your procurement past.
Discover More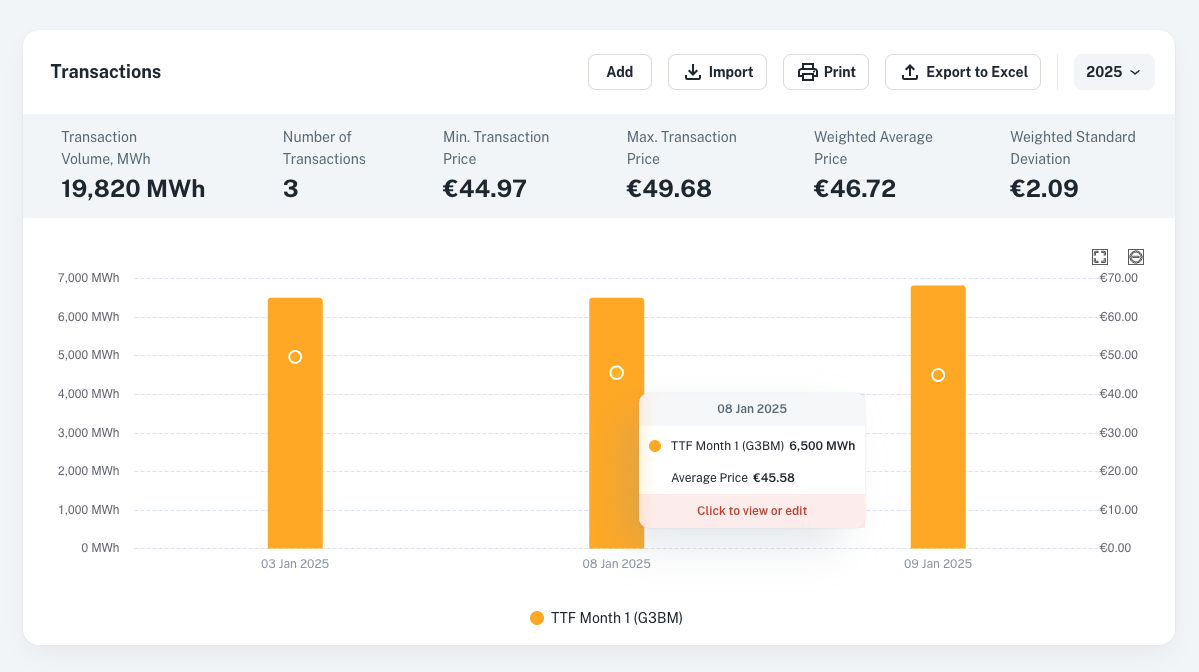
Prices & Volatility : Instant Market Insights
Stay on top of price fluctuations and act with confidence, before volatility impacts your costs.
Discover More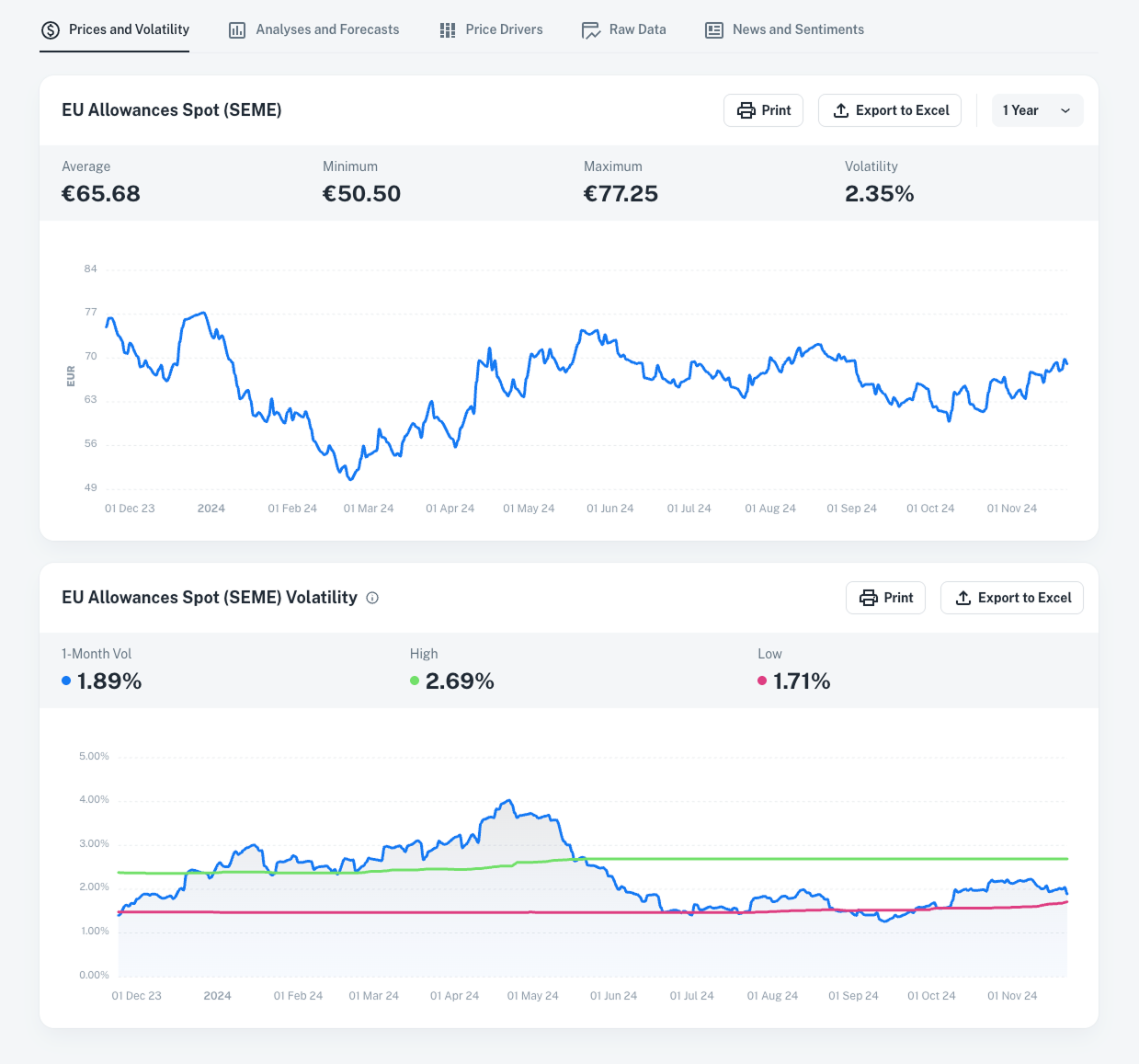
Analyses & Forecasts : AI-Powered Price Projections
Leverage advanced forecasts to align purchasing decisions with future market conditions.
Discover More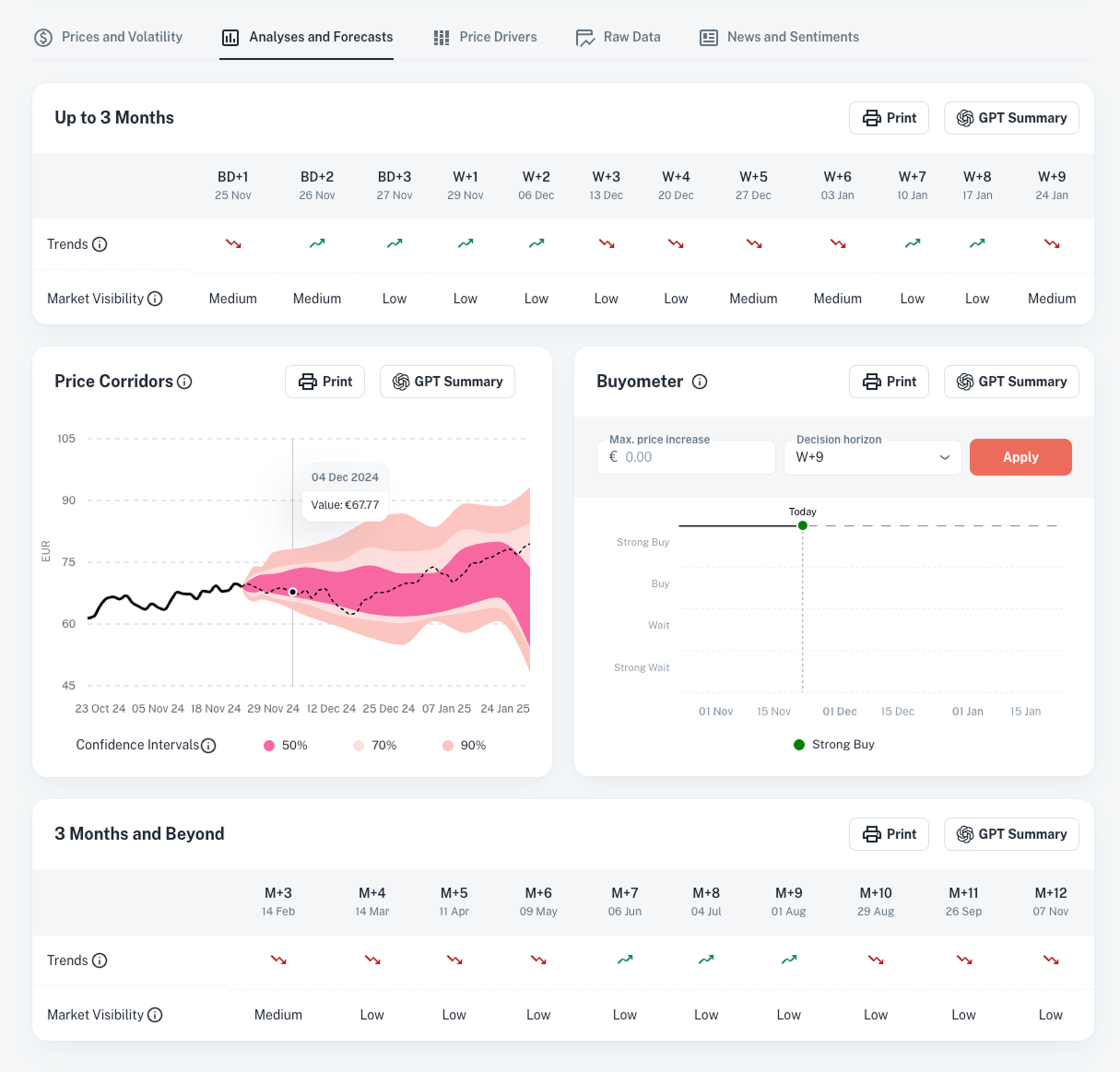
Price Drivers : Understand What Drives Your Market
Identify the variables that matter most to refine your strategy and eliminate data noise.
Discover More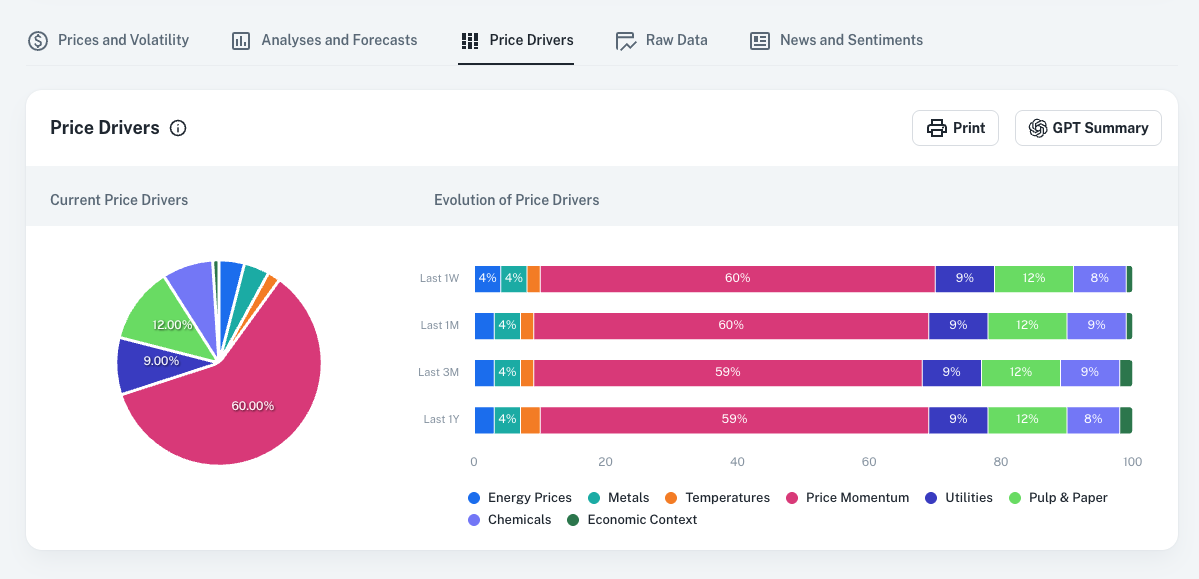
Data Stream Selection : Optimize Your Market Intelligence
Choose only the most relevant data inputs to reduce complexity and control data costs.
Discover More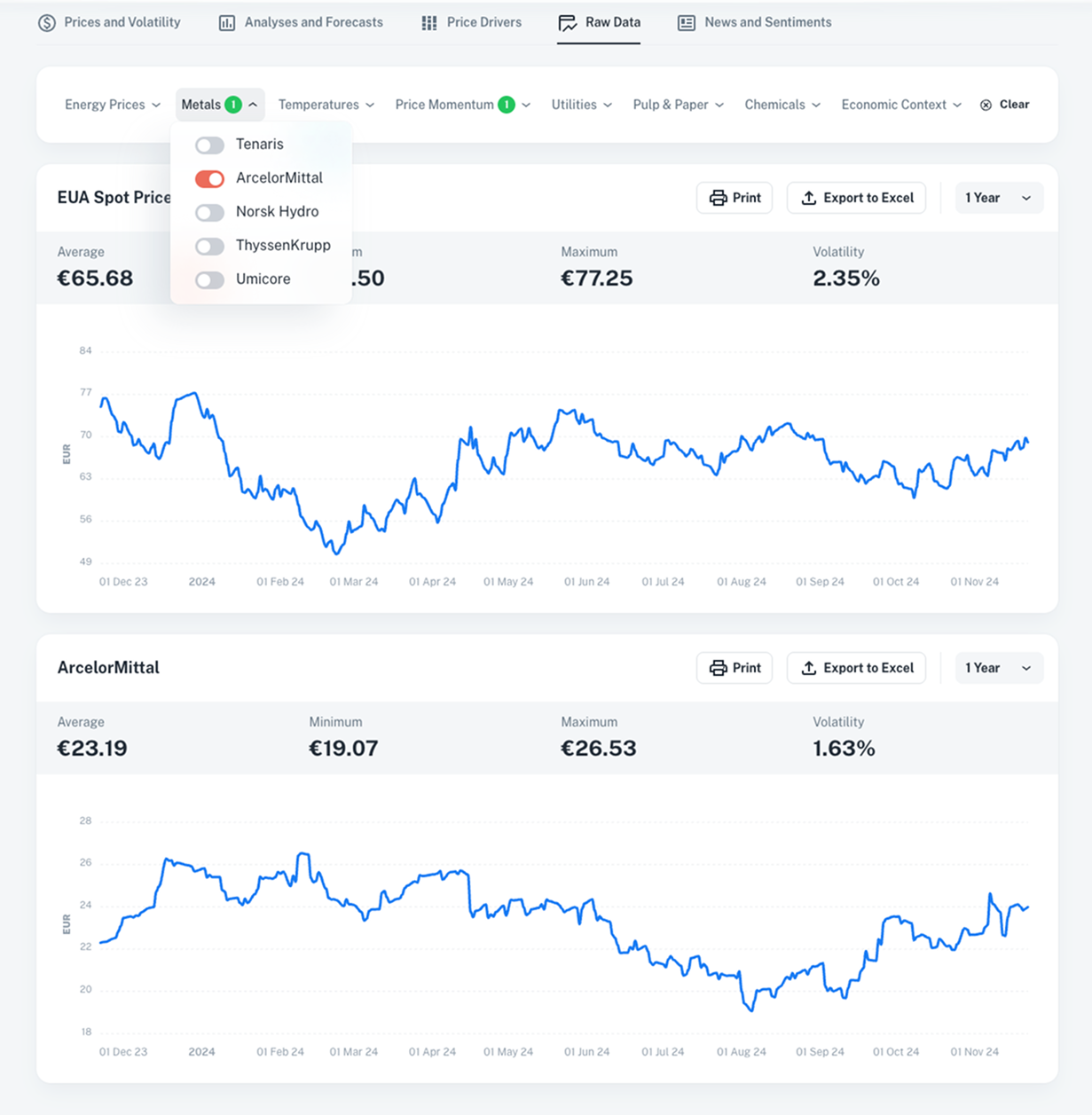
Configuration : Design & Test Multiple Strategies
Explore different purchasing and hedging scenarios in a risk-free environment to find what works best.
Discover More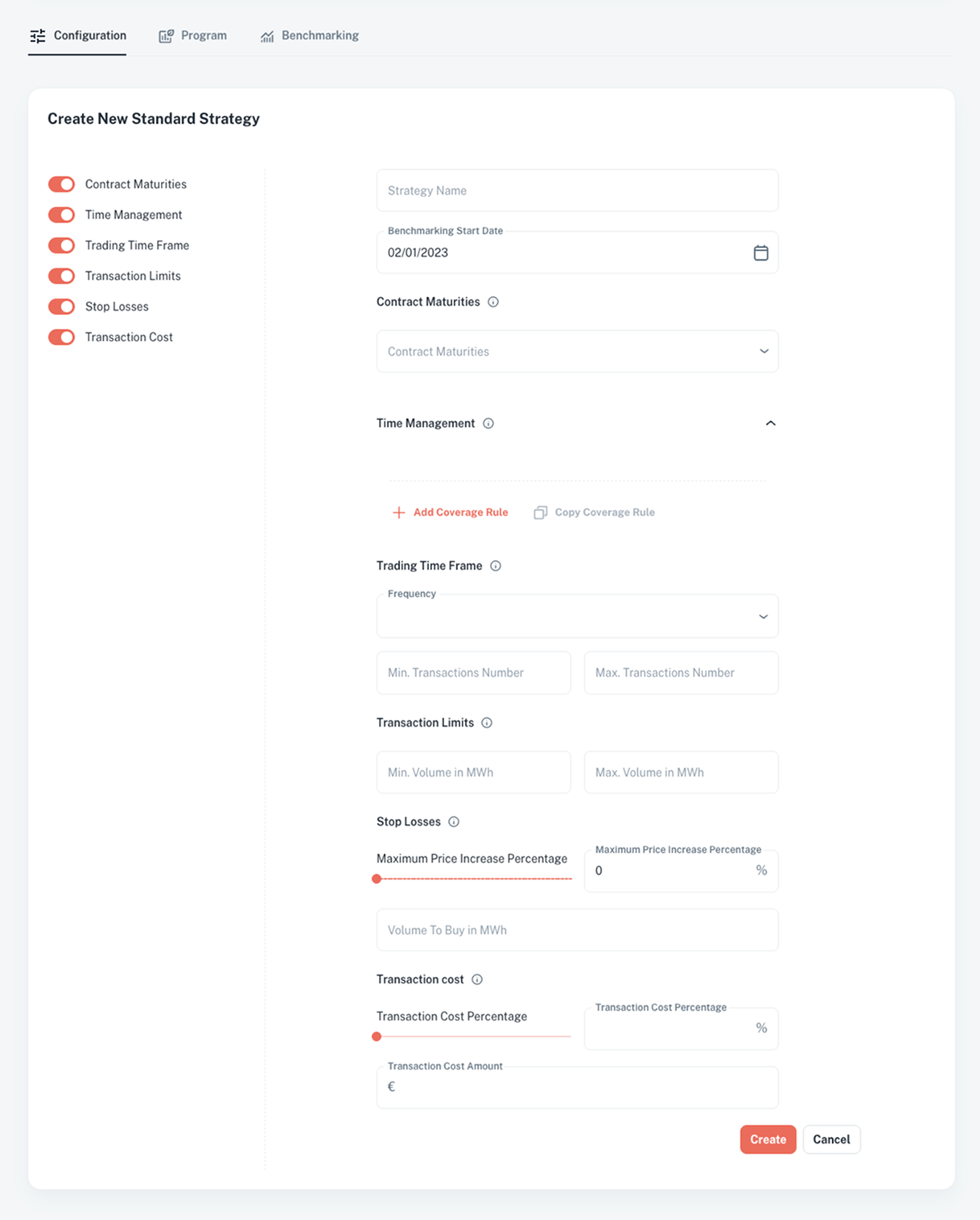
Program : AI-Powered Strategy Execution
Execute AI-enhanced decisions while keeping full ownership, and reduce your procurement costs by up to 5%.
Discover More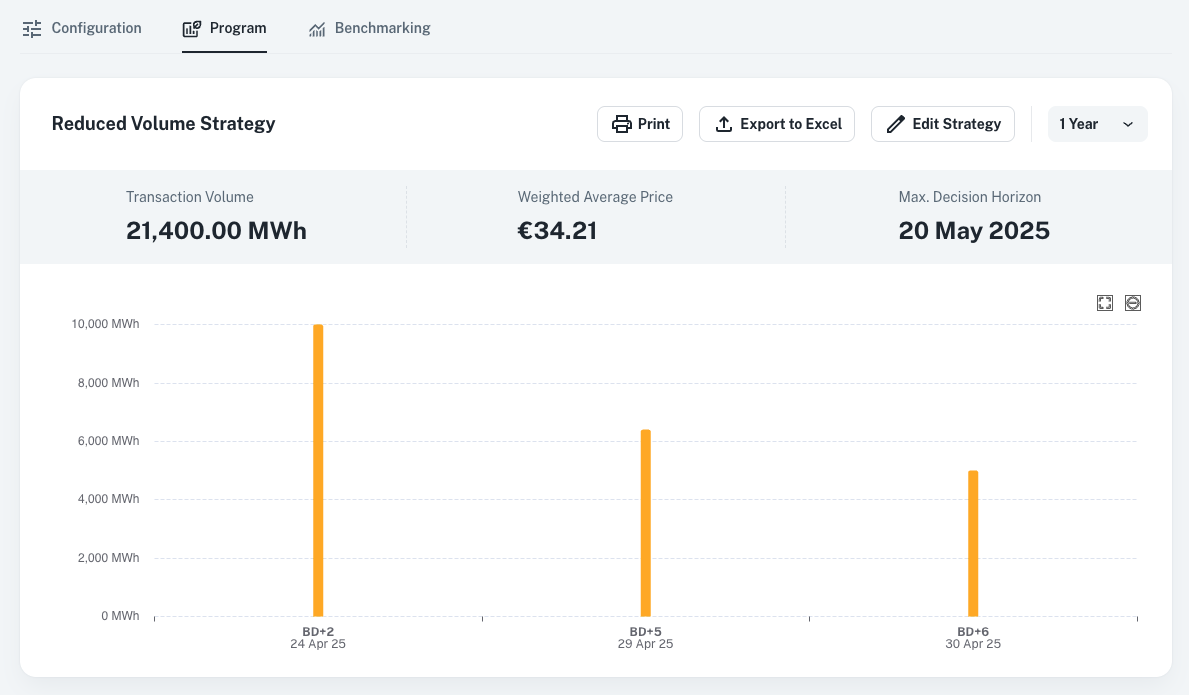
Benchmarking : Compare & Optimize Performance
Compare the impact of your different strategies over time and continuously optimize performance.
Discover More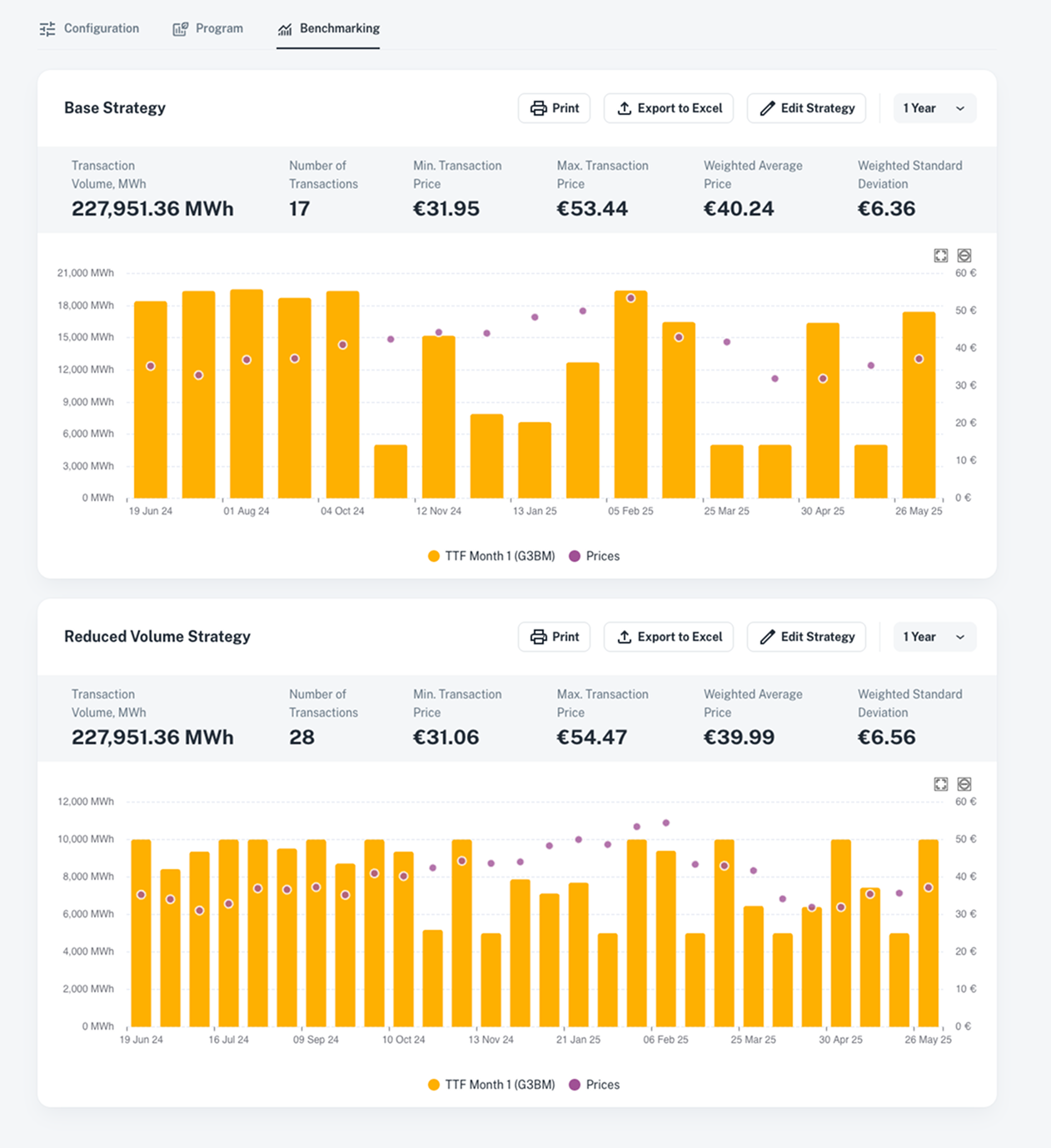
Take the next step
Explore the benefits and value Datapred brings to your company.
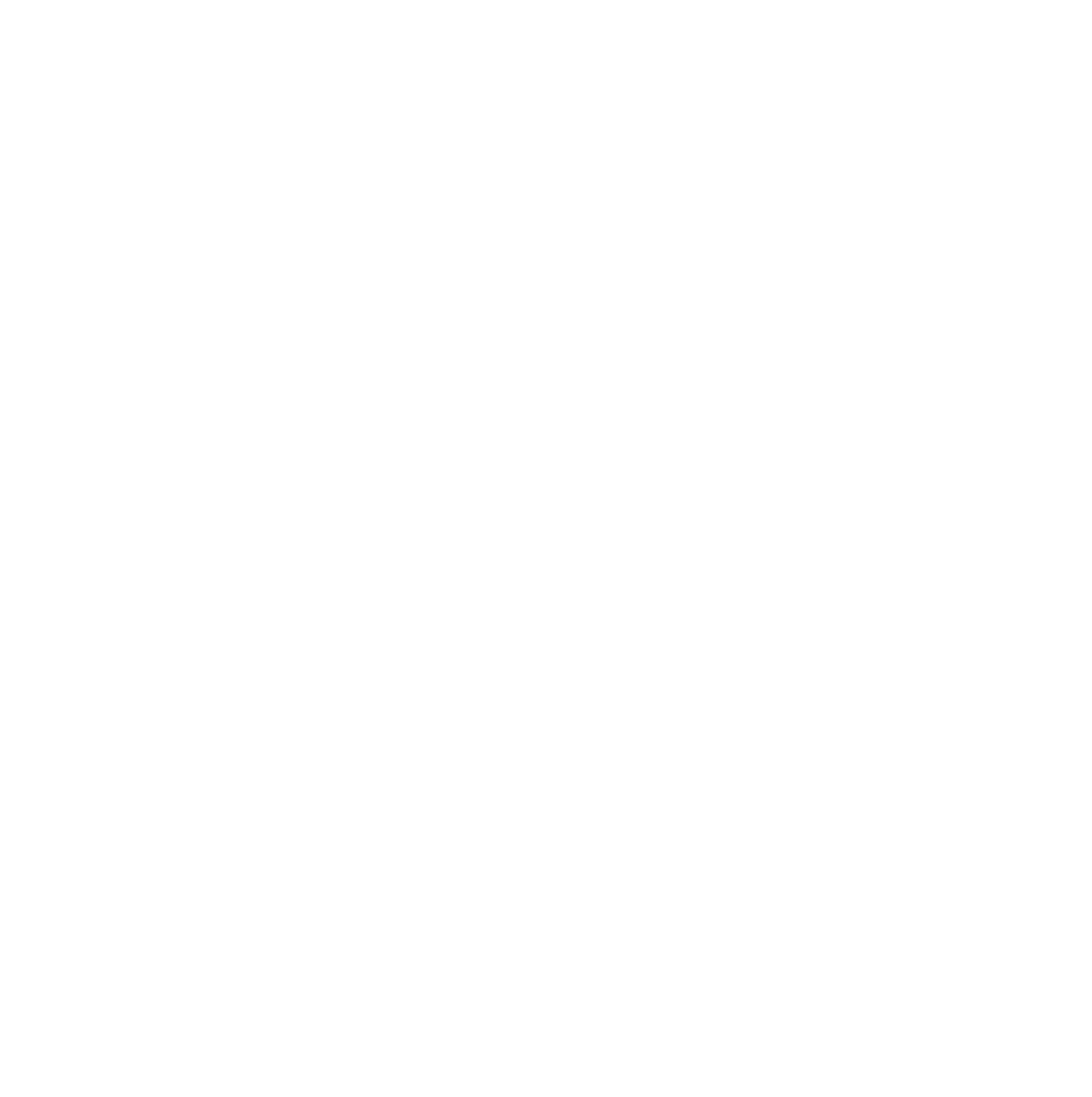